Ed Challis, Head of AI Strategy at UiPath, recently led a roundtable discussion with Crew Capital portfolio company founders and technical leaders. During the conversation, Ed provided thoughtful perspectives around practical applications of AI within startups and enterprises, the evolving complexities of ML systems, and how founders can make informed decisions around trade offs when building AI-driven products. Here are some of the key takeaways from our discussion.
The importance of training data in AI systems
Ed’s career trajectory provides a unique perspective on the challenges and opportunities AI startups face. After a stint in investment management, he went on to earn a PhD in Machine Learning. Ed then co-founded Re:infer, a startup that leveraged AI to help enterprises gain context from customer interactions by extracting insights from channels such as emails, calls, and notes. Reflecting on his early experiences, Ed emphasized the critical importance of collecting the highest-quality training data possible for machine learning models.
“It’s my strong belief that, at least 95% of the time, companies can’t get complex ML systems to work because they don’t have the right training data,” he said. “If data analysts could make the process of collecting context-rich training data easier, it would be easier to address problems around pattern identification, forecasting, and other model outputs more effectively.”
Ed shared that his early research revolved around deep learning and natural language processing, specifically focusing on how AI could generate actionable insights from conversational data. After months of speaking with enterprise leaders to understand their sharpest pain points in growing their businesses, Ed identified the intersection of AI and automation as a key opportunity to build a solution to contextualize the voice of the buyer beyond historically manual processes of reviewing call notes and documents exchanged.
Implementing evaluation frameworks
One of the core themes Ed discussed was the necessity for engineers to leverage robust evaluation frameworks when developing AI systems from the ground up. Building effective machine learning models requires a deep, iterative approach to testing and evaluation, especially given the inherent uncertainties in the process.
“No matter how strong your intuition about a particular model or approach, the truth is always more elusive and intricate,” Ed said. “Often, you’ll have a strong hunch that some technology will work well, and then it just doesn’t. Engineers need to be nimble, especially in the foundational stages of building out new systems.”
He stressed that evaluation is not just limited to checking a model’s accuracy against benchmarks, but moreover understanding and managing the inherent randomness within machine learning systems, and its sources. This randomness can arise from several different sources—training data, test data, and production data—and developers must constantly iterate on their evaluation frameworks to adapt.
“Developers can never be 100% confident if something’s going when deploying a new system,” he said. “The only thing they can control is to create a solid evaluation set, come back to it again and again, and continually improve it in order to generate predictable and accurate model output. That’s how to uncover repetitive failure and figure out what works in practice.”
In recent years, fine-tuning has grown in popularity as a technique for improving model performance when directed at specific tasks. Ed highlighted that while this can improve accuracy in specific scenarios, he believes that rigorous, ongoing evaluation processes ultimately are what ensure a model’s efficacy in production.
“Fine-tuning might get you important advancements in the short term, but without a robust evaluation process, you’re just guessing,” he said. By focusing on a structured approach to data evaluation at each step, startups can better navigate the complexities and surprises around product development, ensuring their models deliver reliable results.
Balancing approaches to optimizing model outputs
Ed shared his perspective on fine-tuning versus retrieval-augmented generation (RAG), an innovative approach in AI that merges the precision of conventional information retrieval methods, similar to those used in database systems, with the creative and linguistic abilities of large language models (LLMs).
Ed cautioned against relying on fine-tuning as a silver bullet when improving AI models. “Fine-tuning can increase a model’s ability to perform a certain set of tasks, but I see it more as a way to sharpen focus on specific attributes or problems, it is less general-purpose,” Ed said. “It’s important to remember that using RAG techniques to pull in relevant information for answering specific questions might be a more efficient approach based on what you’re solving for.”
In fact, Ed underscored the importance of focusing on end-to-end system evaluation rather than fixating on a single model or technique. For startups, this means applying a methodical approach to AI development: Start with simpler models, establish a solid evaluation framework, and then explore model-specific optimizations. “Developers need to think about the whole system they are building,” Ed said. “It’s not just about improving the model, but also the product it ultimately drives. Products need to deliver value even when the model doesn’t perform perfectly.”
The role of smaller models in regulated industries
When asked about the relevance of smaller models within the current AI landscape, Ed acknowledged their value amongst companies in regulated industries or with limited computational resources. However, he pointed out that the decreasing cost of running large models has shifted the equation for many startups.
“If you’re thinking about big models and small models purely from a cost perspective, it’s an interesting variable to be aware of,” Ed said. “Big models are getting cheaper by orders of magnitude, but there are still good reasons to use smaller models — especially in environments with significant compute constraints and industries that still do the majority of deployments on-prem.”
In regulated industries, particularly in regions like Europe, navigating complex legal frameworks around data privacy and collection can present significant challenges. These regulations, including the General Data Protection Regulation (GDPR), demand strict compliance and can limit how companies collect, store, and use data for training AI models. For many startups, this means balancing the need for innovation with the reality of legal scrutiny. Ed emphasized the importance of getting data compliance right from the outset.
“I’m based in Europe, and one of the biggest problems around data collection is navigating the evolving sea of regulations. Getting all your ducks in order there is is really, really important. As a startup, there’s not much oversight, and you could end up collecting data incorrectly. But ultimately, if you’re successful, how you collected that data will eventually come under serious scrutiny,” he said.
Ed also noted that smaller models might be particularly useful when latency is a concern, but for most startups, he recommended exploring larger models where possible, given their increasing accessibility and potential for higher performance at scale.
Building collaborative teams in the AI era
When it comes to building a high-performing startup environment, Ed stressed the importance of forming tight-knit, cross-functional teams that blend technical expertise with product management and design.
“I’m a huge believer in teams that have all the necessary skills in one tight pod,” he said. “Startups need a back-end developer, front-end developer, designer, product manager, and machine learning engineer working closely together to build compelling AI-first products. Having all these perspectives in the same room helps ensure you’re building something that’s not just technically sound, but also customer-centric.”
Ed also touched on the need for domain knowledge within AI teams, particularly when building industry-specific products. He emphasized the value of having engineers and data scientists who understand the business problems they are solving, which can significantly improve the relevance and performance of the AI solutions being built.
The future of AI and automation
Looking ahead, Ed remains excited about the potential of AI to transform industries, particularly as the technology becomes more sophisticated and accessible. He shared that his team at UiPath is working on multimodal generative AI models, which combine text generation with actions, enabling more complex workflows to be automated across all industries.
“We’re training our own generative models that can not only generate text but also take actions as part of the automation process,” Ed said. “There’s so much potential in this space, and we’re still figuring out the best ways to harness it.”
In closing, Ed reiterated the importance of staying agile and open to new approaches as the AI landscape rapidly evolves. “Everyone is still figuring out the best way to use this technology. The key is to keep experimenting, keep evaluating, and stay focused on delivering real value to your customers.”
Related Articles
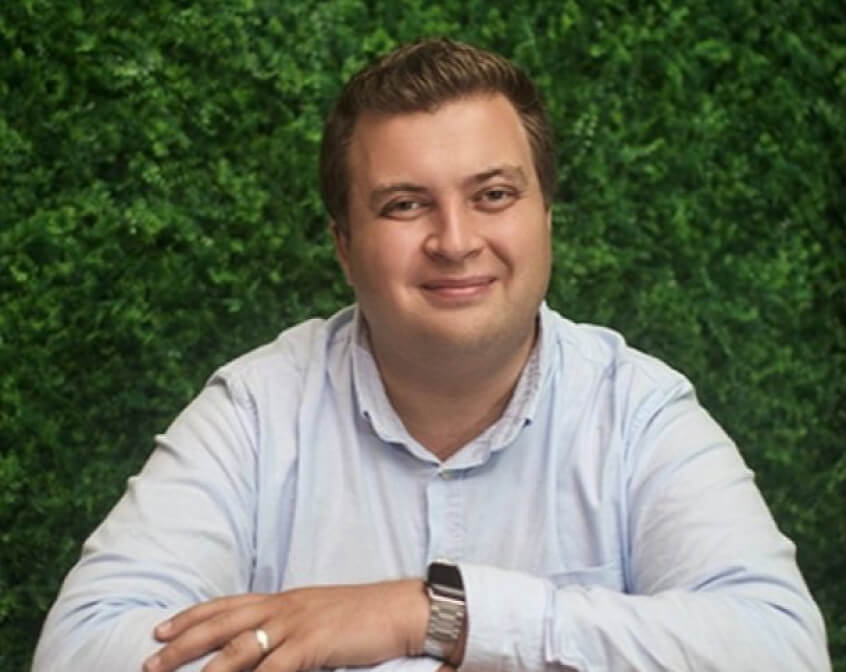
Chris Turlica: Scaling Culture through Hyper Growth
We recently sat down with Chris Turlica, the founder and CEO of MaintainX, to talk about how he and his…
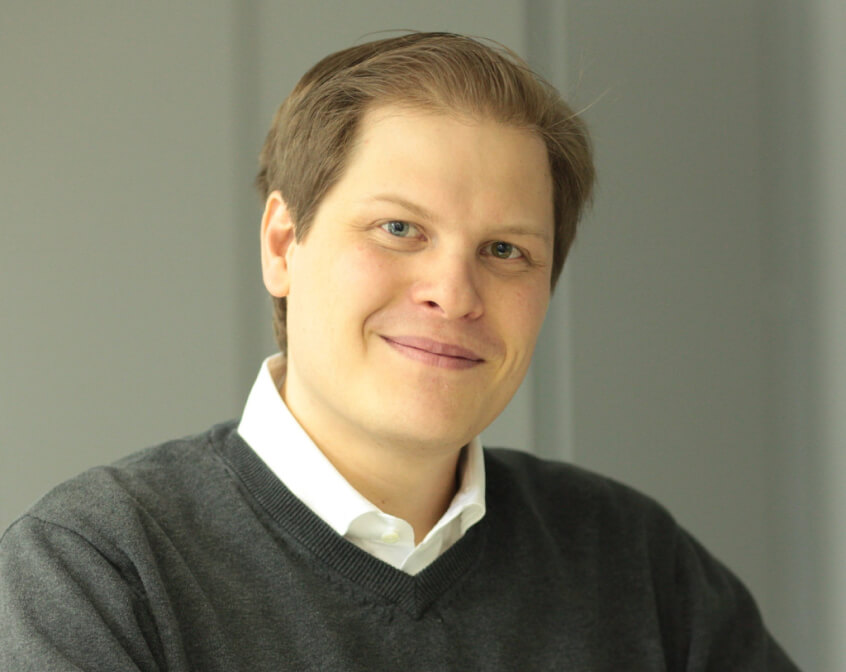
Bene Sauter: Customer Education & Redefining the ERP Market
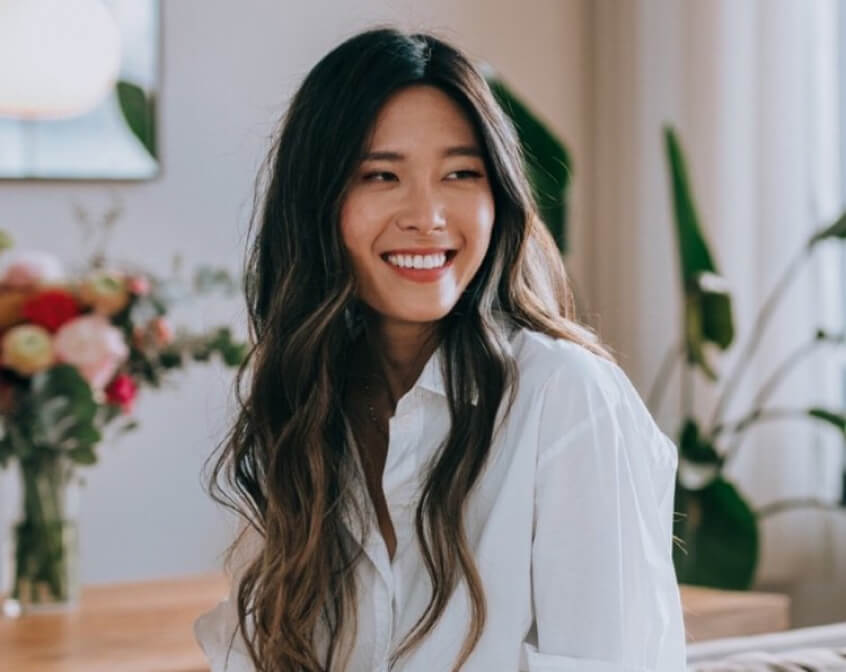
Building a Mental Health Unicorn:
The Entrepreneurial Journey of April Koh
As part of our founder interview series, Crew Capital’s Dylan Reider and Sonia Damian sat down with April to discuss…